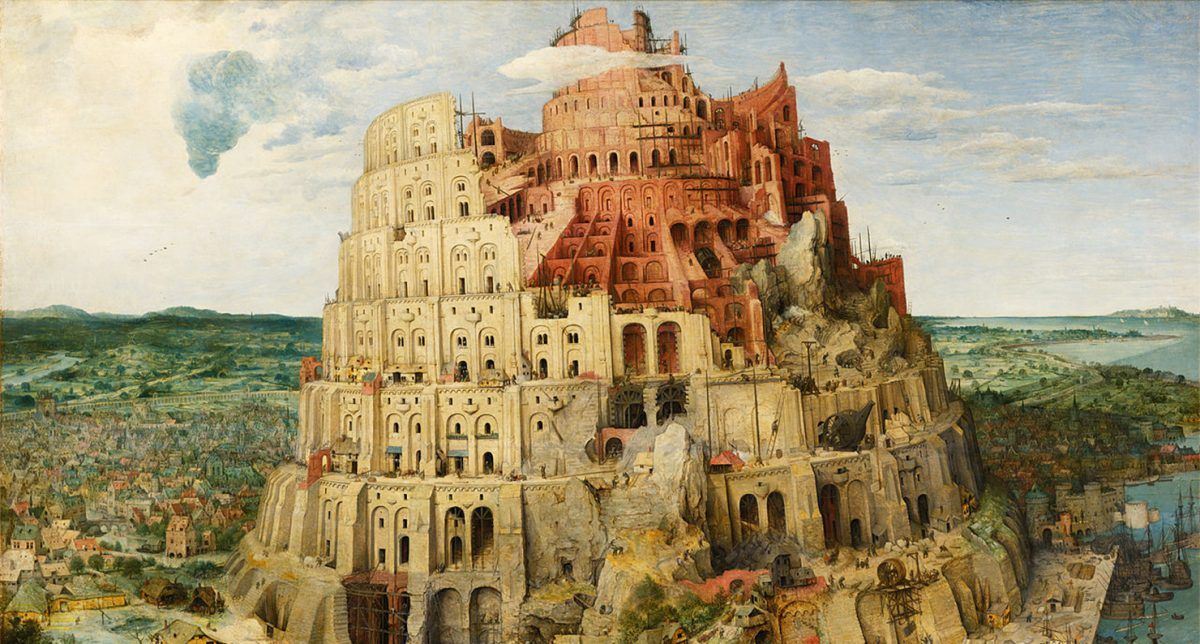
There is an astounding amount of new text produced every day. Millions of daily news articles, blog posts, and social media make up a vast trove of documents that would take the average person a lifetime to read. How can anyone stay on top of this river of new information? More importantly, how can you separate the signal from the noise, focus on the information that matters and ignore the rest? The team at Primer AI, a 75-person startup based in Silicon Valley, believes artificial intelligence is the answer. They crafted machine learning systems that read through documents at tremendous speed, understand which bits are salient information for a given client, and then produce a report that summarizes key findings. “I’ve been curious for a long time about how we can use algorithms and large scale data to better understand the world around us,” said Amy Heineike, Primer’s VP of Product Engineering and founding team member. Before focusing on the world of language, Heineike was a mathematician and spent years working to design models of large cities, studying how people interact in the economic systems of these urban ecosystems. She enjoyed the work, but was often frustrated by the lack of solid data. The census information she relied on was sparse and not frequently updated. The constant flow of new language pulsing across newswires, financial terminals, and scientific journals felt like a potential gold mine. It wasn't clear at the outset whether they'd be able to generate text as the interface to that information. "In the first couple of years we experimented deeply, and we threw a lot of spaghetti at the wall," Heineike recalls with a laugh. "So basically take a huge amount of text, figure out what's important, and then work out the words to say it. It was not at all clear that it was possible or even the right thing to do. My husband, for the first three months of Primer, thought we should be building charts instead." One of the first clients to approach Primer was In-Q-Tel, a venture capital firm borne out of the CIA that serves as a bridge between high-tech startups and national security agencies. At the time, the team was just five people, but it was leveraging a number of major breakthroughs happening in the worlds of natural language processing (NLP) and natural language generation (NLG). “We put a brief together based on thousands of documents that we scanned over the course of a month. Our system spit back out a report that was easy to read and identified crucial events. Even my husband had to admit it was pretty cool.”
William Du, one of Primer’s data scientists, vividly remembers his first experience with the technology. “I walked in and the interviewer asked me what I wanted to learn about. I picked something random from the news that morning, [which was] North Korea test-firing a missile. The system spit out this one page summary. It had a lot of good detail and was easy to understand. Then we peeked under the hood to see how it worked, and I realized it had analyzed hundreds of thousands of articles in the span of a few minutes.” Building a summary is harder than it sounds. “When you look at some of the cutting edge techniques in this field, they are amazing when they work, but then sometimes you get complete gibberish,” explains Du. “So our challenge is to find an algorithm that strikes the right balance between stability and sophistication.” In his recent work, Du has been challenged with applying models to streams of evolving content. The algorithms need to classify documents even when they introduce new terminology. “The future that we're hoping to push for is a representation that will allow for new words to come in, and the model can still derive meaning from a document, even if it doesn't have the specific semantic meaning for a word.” Document representations enable the company's algorithms to perform well on a range of tasks like classification, summarization, and named entity resolution. “As we move forward, I think a big thing that I'm really excited about, and what the whole company is really excited about, is how do we keep pushing forward in this new world of deep learning and deep representations?”
After starting out in the world of national security, Primer has expanded to clients in the legal and financial markets. Hedge funds and banks leverage scans of press releases and regulatory filings for data they can use to trade. Additionally, law firms often have to wade through millions of pieces of documentation when preparing for a trial, even when just a few pages contain information pertinent to the case. Primer’s system can help to speed up the discovery, analysis, and generation of legal reports. Anna Venancio-Marques, a senior data scientist and engineering manager at Primer, came to the world of tech startups from a PhD in Chemistry. “Right now, on the academic side of things, NLP is just bursting with activity. In 2018, there were a lot of models that were developed that are pretty revolutionary,” Venancio-Marques explains. “One of the big names is BERT from Google. They're able to do a lot of the standard tasks at levels we’ve never seen in the past. The industry is tracking academia very closely and we are currently working at bringing BERT models into our production systems.” For a long time, the biggest breakthroughs in deep learning systems were centered on image recognition, but Primer and others believe that is changing. “We're seeing a lot of the learning from images come to text. Transfer learning is one of them, learning on one set of problems, and transferring it to another. It's really exciting that we get to do that in natural language processing as well,” says Venancio-Marques. “It's been working wonders in the image world, so getting into the text world is fantastic.” To learn more about Primer, check out their blog or listen to their CEO in this video. To learn more about how Primer has been using Stack Overflow for Teams to quickly scale and accelerate their software development process, check out this case study.