Since the AI floodgates opened with the release of ChatGPT in Nov 2022, the industry has grown and expanded to cover nearly every tech company today. According to Crunchbase, a little over $100 billion was invested into AI and AI companies. While many of you have been vocal about being tired about hearing about AI, it’s clear that the folks with the money aren’t getting tired of investing in it.
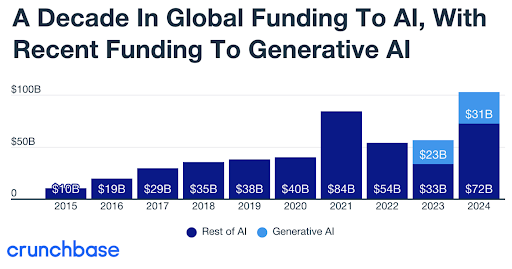
On the podcast, I talk to people every week who are finding a niche for genAI, whether that’s security testing, healthcare streamlining, or API management. It’s still a very new tech, and builders are hard at work finding ways to use AI to solve real world problems, not just flood the web with slop. In March, I went to the HumanX conference to catch up with what problems AI is being used to address.
Before the conference, HumanX shared a report that they produced in association with Crunchbase that details where the money is going in the AI world. On top of that, they used Crunchbase’s AI-powered Prediction Engine to predict what’s next, and what’s next is more funding and more acquisitions. Their AI engine predicts that nearly 30% of the companies on stage at HumanX may be targets for acquisition in the next year.
At the conference, I had the chance to talk to the CEOs of both HumanX and Crunchbase, as well as others, about the state of the AI sector to see if this influx of money and the rollups that are happening are a good sign or an indicator that the AI hype train is finally coming off the rails.
From greenfields to enterprise
I started my career in tech in the early days of the web and saw the money flow in for everybody who knew how to close an anchor tag. At the time, it was great—the money trickled down in amenities, cool offices, and pay. But the bubble burst in 2001. Anyone who’s been in tech a while gets a little nervous when the money starts piling on to a new tech; it looks like another bubble.
Sometimes a bubble doesn’t burst with a fire sale on foosball tables and empty Silicon Valley office space. Sometimes it looks like a maturing of the space as big players consolidate their position and field tested use cases find market fit. The path for startups isn’t binary—it’s not unicorn or die. Plenty of startups find a soft exit in an acquisition. We saw plenty of consolidation last year, and will likely see more this year as genAI and its components commodify.
Last year, there were lots of AI acquisitions: Nvidia bought Run:ai and OctoAI, Databricks acquired MosaicML, ServiceNow picked up Moveworks, and more are happening every day. In the report mentioned above, Crunchbase indicates that 45 of the 153 private companies at HumanX (29%), are very likely to be acquired. For a couple of decades, startups chasing unicorn status often took an acquisition as a pretty good alternative outcome. But the speed at which companies go from startup to exit seems blazingly fast—ChatGPT only broke the GenAI market open at the end of 2022, a mere two and half years ago.
“Consolidation's natural in an early market,” said Stefan Weitz, CEO of HumanX. “I got a great stat from Primary Ventures: in the turn of the 1900’s, there were 2,000 car companies and over 3,000 unique car models. So as an investor, if you're there in 1904 and you're looking at this, would you have picked Ford? Maybe. We've seen it over and over again in the internet and early internet days, but the thing that surprised me the most was that number of companies that could be rolled up within a year, a year and a half of instantiation.”
In an early market, the possibilities seem endless and everybody wants a piece. It’s a state of irrational exuberance, where investors make big bets with long odds in the hopes of hitting the jackpot. In a conversation with Tomasz Tunguz of Theory Ventures at HumanX, he talked about the bubble economy as being a feature, not a bug. “There's this wonderful book by Carlota Perez. She talked about massive innovation cycles, and she breaks it down into the installation and commercialization phase. Installation is the deployment of the railroads, the fiber optic cables, telephony systems, and there you need irrational exuberance to finance hugely capital intensive projects whose ROI is totally uncertain and very likely to be negative. Then you have a correction and software companies are built. It's just part of the boom-bust cycle that we love.”
It’s not just just the venture capitalists that are looking for the next big thing; the current big things want to get a piece of the innovations that may be happening. “We see a lot of these large corp dev departments hunting on Crunchbase trying to figure out which companies they should acquire,” said Crunchbase CEO Jager McConnell. “These companies are desperate to figure out what innovation they should have internally. They've got roadmaps that are out three years, and that's just too slow. They see this little point of ingenuity, this amazing little piece, and they want to bring it in.” Tunguz agrees: “The cost of missing a company is much greater than the cost of investing in a company that does not work.”
For the companies doing the innovation and getting rolled up, they are betting on winning the lottery as well. With irrational exuberance, tons of entrepreneurs come up with similar ideas, but really, only one or two will hit paydirt. “I don't know how any normal human being without being an AI could actually figure out which companies could solve their needs,” said Weitz. “There is just cacophony out there; to break through and grab attention is difficult and in most cases will not happen.”
With that climate, as we see more and more AI startups jump in, founders and their investors may start looking for ways to get some return on their money. “It's like, ‘Let's take the money when we can because we don't know what tomorrow's going to bring,’” said McConnell, roleplaying a VC. “There are obviously great exits that are happening. I think investors are happy to take that win especially after many years of losses.”
Disrupting the disruptors
Bubble or not, AI has certainly made some waves, and everyone is looking to find the right strategy. It’s already caused a great deal of disruption—good and bad—among software companies large and small. The speed at which the technology has moved from its coming out party, has been stunning; costs have dropped, hardware and software have improved, and the mediocre version of many jobs can be replicated in a chat window. It’s only going to continue. “AI is positioned to continuously disrupt itself, said McConnell. “It's going to be a constant disruption. If that's true, then all of the dollars going to companies today are at risk because those companies may be disrupted by some new technology that's just around the corner.”
First up on the list of disruption targets: startups. If you’re looking to get from zero to market fit, you don’t need to build the same kind of team like you used to. “Think about the ratios between how many engineers there are to salespeople,” said Tunguz. “We knew what those were for 10 or 15 years, and now none of those ratios actually hold anymore. If we are really are in a position that a single person can have the productivity of 25, management teams look very different. Hiring looks extremely different.”
That’s not to say there won’t be a need for real human coders. We’ve seen how badly the vibe coding entrepreneurs get dunked on when they put their shoddy apps in front of a merciless internet. The marginal cost of good enterprise software might still be significant, but the cost for a small app that you need for a single purpose will likely be close to zero. It doesn’t need to be secure or efficient, it just needs to work. While I don’t think software engineers will be mooted, the labor market for you all will certainly feel some shockwaves. Contractors look to be the first to go.
Next up for disruption: SaaS companies. Those apps that live in a webpage or mobile app may not need to have the same front end they have today. I’ve been half joking on the podcast that every SaaS company is going to become an API. Agents are already pushing to access various SaaS abilities in a programmatic way; the development of Model Context Protocol looks to standardize LLM access beyond just APIs. “What if an LLM just creates the UI that I need for the thing that I'm trying to do?” said McConnell. “Then it does just become a services based model, all APIs everywhere, and the AI just reaches out when it needs to. Can the AI just make the thing so it doesn't need to make the call? That's where you have disruption on those business models.”
Isaac Lyman wrote on this blog that AI was just a UI. That’s coming true in more ways than he wrote about then. Not only are GenAI tools replacing the web searches and sites, but they are also replacing the web interfaces to various web-based tools. “UIs are simply abstractions between human clumsiness or our way of thinking and silicon,” said Weitz. “When I think about what AI can do, it should be one canvas to rule them all. You shouldn't have to think about opening this app to send an email, you should just send the email.” As Tunguz put it: “All those companies were built predicated on a particular workflow. All of those processes are changing. The calcified software over the last 20 years no longer works, which means all of that market cap, one and a half trillion dollars worth, is now loose.”
Finally, the big targets for disruption: the AI companies themselves. There’s two factors coming for them. First, all this investment has created some pretty significant infrastructure. More powerful chips are being designed, better power management created, and processing methods improved. “The inference and the overall execution costs are significantly less than they were at the beginning,” said Tunguz. “I think we've seen a 1,000x in price performance improvement. I think we'll probably see another 1,000x.”
The second is open source. When Deepseek released their reasoning model in January 2025, the industry collectively gasped. Because of export restrictions, it wasn’t trained on top-of-the-line GPUs and matches OpenAI’s o1 model on certain benchmarks while costing 96% less in production. Nvidia’s stock dropped 17% on the news. Plus, you can download the model for free right now and do as you like with it.
There are big questions about the model training, like how much distillation was there? That’s where a model trains off the responses of another model. But that distillation, that remixing and retraining may be part of the process going forward. “When you have a new high watermark that's closed source, how long does it take for open source to come and beat it?” Tunguz asked. “In 2023 it was 140 days and in 2024 it was 41. There's no deep research API that's available aside from Perplexity’s Grok. I think part of this is to forestall the commodification of this next wave of super expensive models.”
With this constant disruption potentially in our future, how does one start or run a business? For that matter, how does one choose a vendor to build a long term solution on? “What's going to drive at least the next five years of customer action is going to be who do I trust, especially at those foundational layers,” said McConnell. “Even if there is a new disruption, do I trust it? That's immediately what happened around DeepSeek. It was like, ‘Oh, well, it's disrupted. It's cheaper. It's so much better. Oh, but I don't trust it. So now I'm going to go back and I'll pay more for the other model.’”
This has been a fundamental tension with open source since software starting eating the world 20, 30 years ago. Open source software has found a home at nearly every modern enterprise, but that wasn’t always the way. “I remember back in the day I was at Microsoft, we couldn't use any open source at the time,” said Weitz. “It was partially because we didn't want to deal with the license, but also because, do you really trust this library?”
In some ways, it may be the old adage of “you get what you pay for.” A business that doesn’t pay anything for software, may get more problems than solutions. We’ve talked to plenty of companies building businesses on top of freely available open source software. By paying a company for open source software, you get services, support, and trust. “People always ask me, ‘Why would anyone do open source?’” said Weitz. “Well, you're not selling the software, you're selling the services, you're selling a wrapper or a hosted version, or all the different things that make sense for a company to make it easy to use.”
There’s one more thing that makes for a killer app in the age of AI disruption: data.
Real solutions are built on good data
In walking around the floor at HumanX and talking to folks for podcasts, it struck me that AI fever has struck all parts of the stack, from custom cloud compute and artisanal supercomputers to applications in health care and physical infrastructure. I even saw one booth with an art project machine that spat out 80s-style advertising from within the uncanny valley. The big names were there, both in the AI space and in technology in general. The wide range and interest is no surprise—plenty of enterprises are still figuring out how to turn genAI into a business plan.
“How do you help enterprises navigate their way through this AI forest and do it in a way with confidence and conviction?” said Weitz. “That's missing right now for most of the enterprises we've spoken with. They don't have a great AI strategy, They've got maybe an idea or 25. I've talked to one company that had 230 pilot projects running at the same time.” In a lot of ways, this sounds like the shotgun-style VC model for investing in a greenfield market; take a lot of gambles and hope one of them pays out.
For existing enterprises, they’re looking for ways to better serve their customers, to give them more features, and get new customers. Let’s not be coy: they’re investing in AI to get more money. “I worked at Salesforce for 11 years, and I heard a lot of customers asking, ‘Can you help me find my next customer?’” said McConnell. “Those are enterprises that are ready for AI, because as a Salesforce product could say, ‘I understand who you sell to. I'm going to go and find customers like that and give you new customers that look like that.’” that is AI ready for the enterprise if you could come up with that tomorrow.” Or, y’know, in 2024.
There’s more AI use cases besides business. People are saving the bees, predicting wildfires, and neutralizing a superbug in days instead of years. “Some of the best thinking that I've seen in AI has come from interdisciplinary and cross-disciplinary conversations,” said Weitz. “I was at TED last year and these Caltech researchers had this realization that so many hospitalizations were caused by infection. Catheters in particular were a huge problem. They gave that problem to an AI and it created a catheter with basically a bunch of teeth on the inside that would prevent the bacteria from making it all the way. He argued that orthogonal thinking is where we get a lot of innovation, but there's probably a lot of things to be mined from even our historical acts.”
The foundational tech, from the infrastructure to the data management to inference stacks and LLMs, are incredible pieces of software that are opening up some fascinating applications. But the really useful applications are building with this tech on data. All of the user cases above, from the businesses to the non-profits, are all data stories. “The AI companies that figure out how to extract value for their customers every customer’s unique proprietary data, that's where things get interesting,” said McConnell. “As a data company, I feel really good, because if you've got proprietary data that no one else has access to, it's very hard to beat me at the game. Data and differentiation in that data that no one else has access to is the holy grail I think in the long run because, no, AI can't make it up, and that feels like a winner.”
Jackpots and potshots
As the continued rise of VC money shows, there’s a business future in AI. Whether it’s one that belongs to the hyperscalers of today, replaces those previous generation’s giants with new one, or creates a cycle of titans rising and falling is yet to be seen. What does seem to be clear is that everybody needs a strategy for AI, even if that strategy entails not adopting AI. Having some sort of special sauce that can’t be easily replicated will guarantee a place in the market. For most AI use cases, that sauce is going to be data. If you have something no else does, it makes it pretty hard to get disrupted out of the market.