How do you fact-check an AI?
Ryan chats with Amr Awadallah, founder and CEO of GenAI platform Vectara about how retrieval-augmented generation (RAG) has advanced, why fact-checking and accurate data are essential in building AI applications, and how Vectara’s Mockingbird model seeks to minimize hallucinations.
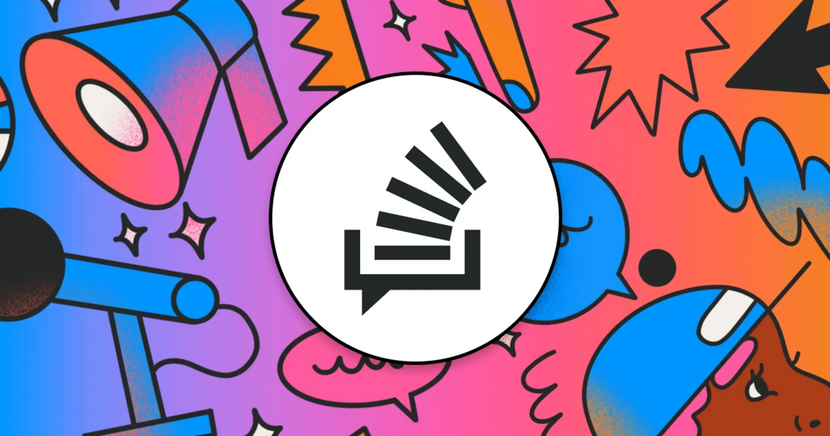