“Translation is the tip of the iceberg”: A deep dive into specialty models
Olga Beregovaya, VP of AI at Smartling, joins Ryan and Ben to explore the evolution and specialization of language models in AI.
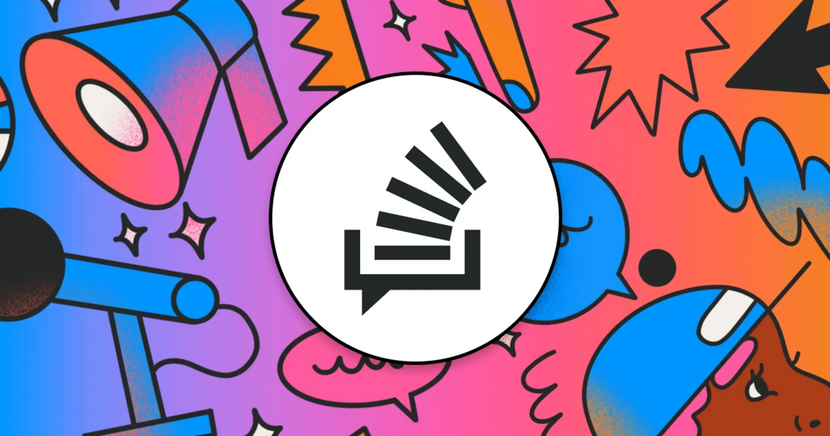
Olga Beregovaya, VP of AI at Smartling, joins Ryan and Ben to explore the evolution and specialization of language models in AI.
Want to train a specialized LLM on your own data? The easiest way to do this is with low rank adaptation (LoRA), but many variants of LoRA exist.
How Diffblue leverages machine learning techniques to write effective unit tests.
A look at some of the current thinking around chunking data for retrieval-augmented generation (RAG) systems.
Wondering how to go about creating an LLM that understands your custom data? Start here.
Ben talks with Eran Yahav, a former researcher on IBM Watson who’s now the CTO and cofounder of AI coding company Tabnine. Ben and Eran talk about the intersection of software development and AI, the evolution of program synthesis, and Eran’s path from IBM research to startup CTO. They also discuss how to balance the productivity and learning gains of AI coding tools (especially for junior devs) against very real concerns around quality, security, and tech debt.
Fabrizio Ferri-Benedetti, who spent many years as a technical writer for Splunk and New Relic, joins Ben and Ryan for a conversation about the evolving role of documentation in software development. They explore how documentation can (and should) be integrated with code, the importance of quality control, and the hurdles to maintaining up-to-date documentation. Plus: Why technical writers shouldn’t be afraid of LLMs.
Ben and Ryan are joined by Matt Zeiler, founder and CEO of Clarifai, an AI workflow orchestration platform. They talk about how the transformer architecture supplanted convolutional neural networks in AI applications, the infrastructure required for AI implementation, the implications of regulating AI, and the value of synthetic data.
Or Lenchner, CEO of Bright Data, joins Ben and Ryan for a deep-dive conversation about the evolving landscape of web data. They talk through the challenges involved in data collection, the role of synthetic data in training large AI models, and how public data access is becoming more restrictive. Or also shares his thoughts on the importance of transparency in data practices, the likely future of data regulation, and the philosophical implications of more people using AI to innovate and solve problems.
Will prompt engineering replace the coder’s art or will software engineers who understand code still have a place in future software lifecycles?
Here's a (brief) summary of language model finetuning, the various approaches that exist, their purposes, and what we know about how they work.
Ben chats with Shayne Longpre and Robert Mahari of the Data Provenance Initiative about what GenAI means for the data commons. They discuss the decline of public datasets, the complexities of fair use in AI training, the challenges researchers face in accessing data, potential applications for synthetic data, and the evolving legal landscape surrounding AI and copyright.
Masked self-attention is the key building block that allows LLMs to learn rich relationships and patterns between the words of a sentence. Let’s build it together from scratch.
Ben chats with Gias Uddin, an assistant professor at York University in Toronto, where he teaches software engineering, data science, and machine learning. His research focuses on designing intelligent tools for testing, debugging, and summarizing software and AI systems. He recently published a paper about detecting errors in code generated by LLMs. Gias and Ben discuss the concept of hallucinations in AI-generated code, the need for tools to detect and correct those hallucinations, and the potential for AI-powered tools to generate QA tests.
Ben and Ryan talk to Scott McCarty, Global Senior Principal Product Manager for Red Hat Enterprise Linux, about the intersection between LLMs (large language models) and open source. They discuss the challenges and benefits of open-source LLMs, the importance of attribution and transparency, and the revolutionary potential for LLM-driven applications. They also explore the role of LLMs in code generation, testing, and documentation.
The decoder-only transformer architecture is one of the most fundamental ideas in AI research.
Retrieval-augmented generation (RAG) is one of the best (and easiest) ways to specialize an LLM over your own data, but successfully applying RAG in practice involves more than just stitching together pretrained models.
Ben and Eira talk with LlamaIndex CEO and cofounder Jerry Liu, along with venture capitalist Jerry Chen, about how the company is making it easier for developers to build LLM apps. They touch on the importance of high-quality training data to improve accuracy and relevance, the role of prompt engineering, the impact of larger context windows, and the challenges of setting up retrieval-augmented generation (RAG).
In this episode, Ben chats with Elastic software engineering director Paul Oremland along with Stack Overflow staff software engineer Steffi Grewenig and senior software developer Gregor Časar about vector databases and semantic search from both the vendor and customer perspectives.
Here’s a simple, three-part framework that explains generative language models.
Ben and Ryan talk with Vikram Chatterji, founder and CEO of Galileo, a company focused on building and evaluating generative AI apps. They discuss the challenges of benchmarking and evaluating GenAI models, the importance of data quality in AI systems, and the trade-offs between using pre-trained models and fine-tuning models with custom data.
The home team talks about the current state of the software job market, the changing sentiments around AI job opportunities, the impact of big players like Facebook and OpenAI on the space, and the challenges for startups. Plus: The philosophical implications of LLMs and the friendship potential of corvids.
On this episode: Stack Overflow senior data scientist Michael Geden tells Ryan and Ben about how data scientists evaluate large language models (LLMs) and their output. They cover the challenges involved in evaluating LLMs, how LLMs are being used to evaluate other LLMs, the importance of data validating, the need for human raters, and more needs and tradeoffs involved in selecting and fine-tuning LLMs.
In the wake of the XZ backdoor, Ben and Ryan unpack the security implications of relying on open-source software projects maintained by small teams. They also discuss the open-source nature of Linux, the high cost of education in the US, the value of open-source contributions for job seekers, and what Apple is up to AI-wise.