Is GenAI the next dot-com bubble?
The home team talks about the current state of the software job market, the changing sentiments around AI job opportunities, the impact of big players like Facebook and OpenAI on the space, and the challenges for startups. Plus: The philosophical implications of LLMs and the friendship potential of corvids.
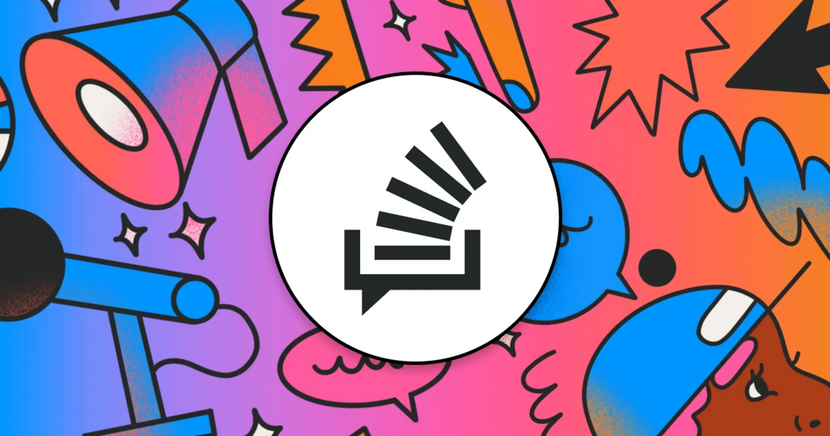