A student of Geoff Hinton, Yann LeCun, and Jeff Dean explains where AI is headed
Ben and Ryan are joined by Matt Zeiler, founder and CEO of Clarifai, an AI workflow orchestration platform. They talk about how the transformer architecture supplanted convolutional neural networks in AI applications, the infrastructure required for AI implementation, the implications of regulating AI, and the value of synthetic data.
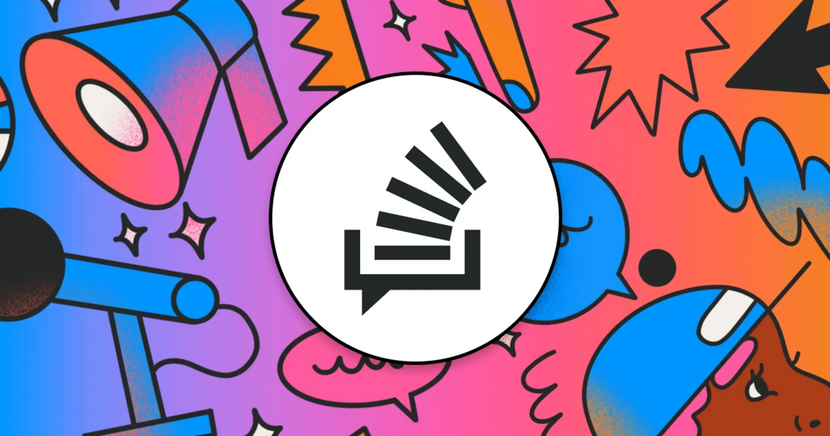