“The power of the humble embedding”
Ryan speaks with Edo Liberty, Founder and CEO of Pinecone, about building vector databases, the power of embeddings, the evolution of RAG, and fine-tuning AI models.
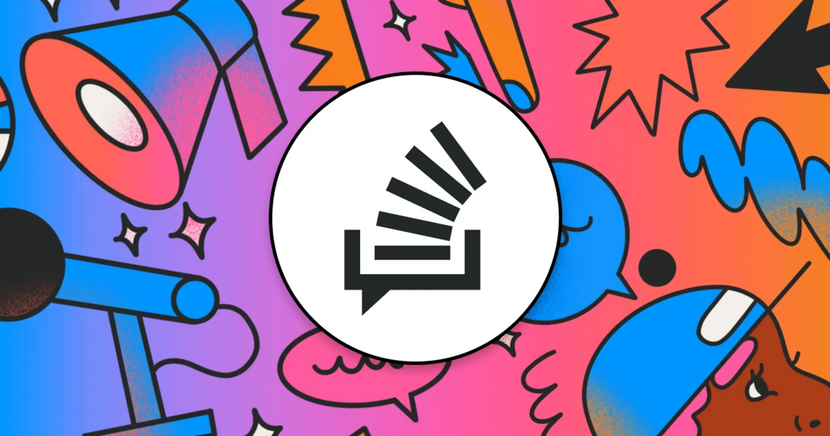
Ryan speaks with Edo Liberty, Founder and CEO of Pinecone, about building vector databases, the power of embeddings, the evolution of RAG, and fine-tuning AI models.
Olga Beregovaya, VP of AI at Smartling, joins Ryan and Ben to explore the evolution and specialization of language models in AI.
Ben and Ryan talk with Geoffrey (Jef) Huck, a software developer turned public speaking coach, about the importance of soft skills in the tech industry—in particular, speaking and communication skills. Their conversation touches on how Huck’s experiences with anxiety shaped his efforts to become a better communicator, practical techniques for dispelling anxiety and connecting with the audience, and the MVP approach to public speaking.
Ryan talks with Sterling Chin, a senior developer advocate at Postman, about the intersection of APIs and AI. They cover the emergence of AI APIs, the importance of quality APIs for AI integrations, and the evolving role of GraphQL in this new landscape. Sterling explains how some organizations are shifting toward an API-first development approach and talks about the future of data access in the agentic era, where APIs will play a crucial role in AI interactions.
In this episode, Ben and Ryan sit down with Inbal Shani, Chief Product Officer and Head of R&D at Twilio. They talk about how Twilio is incorporating AI into its offerings, the enormous importance of data quality in achieving high-quality responses from AI, the challenges of integrating cutting-edge AI technology into legacy systems, and how companies are turning to AI to improve developer productivity and customer engagement.
Ben and Ryan are joined by Matt Zeiler, founder and CEO of Clarifai, an AI workflow orchestration platform. They talk about how the transformer architecture supplanted convolutional neural networks in AI applications, the infrastructure required for AI implementation, the implications of regulating AI, and the value of synthetic data.
The home team chats about machine learning and its applications beyond the hot topic of GenAI, what it means for models to unlearn data, the future of open source, and new frontiers in game development.
Ben and Ryan talk with Jonathan Frankle and Abhinav Venigalla of MosaicML, a startup trying to make deep learning and generative AI efficient and accessible for everyone.
The home team talks with Jaclyn Rice Nelson, cofounder and CEO of Tribe AI, about the explosion of hype surrounding generative AI, what it’s like to work at a startup after working at Google, and how Tribe is leveraging the power of a specialist network.
Hear how Intuit is using AI to help its dev teams ship faster.
Machine learning uses data structures that don't always resemble the ones used in standard computing. You'll need to process your data first if you want efficient machine learning.
Statistically-relevant data, but not actually exploitable.
Curation at scale needs to process a lot of data with a good algorithm.
Serial entrepreneur Varun Ganapathi joins the home team for a conversation about the intersection of physics, machine learning, and AI. He offers some recommendations for developers looking to get started in the ML/AI space and shares his own path from academia to entrepreneurship.
On this episode, we talk to John Myers, CTO and cofounder of Gretel, a company that provides synthetic data for training machine learning models without exposing any of their customers personally identifiable information.
Deep learning models still need testing, but many of the common testing approaches don't apply. But with the right methods, you can still make sure your pipeline produces good results.
Across alien epics and procedural crime dramas, detectives and truth seekers have repeated the mantra: zoom and enhance. It’s passed into popular culture as a much-beloved meme, but in recent years, machine learning has increasingly made this fiction trope into an accessible reality. And we've got the demo to prove it.
The goal of building a machine learning model is to solve a problem, and a machine learning model can only do so when it is in production and actively in use by consumers. As such, model deployment is as important as model building.